It sounds almost too good to be true: the forager operator takes a few photos of the chopped material and is able to clarify the machine is achieving the best chop quality and a high CSPS score for the customer.
KEEPING IT BRIEF
- The Claas chop quality analysis app evaluates the degree of size reduction of the maize kernels.
- Artificial intelligence uses images to recognise the shape, colour and texture of the kernels.
- With a smartphone app, the analysis is easy to carry out.
Around 10 years ago, Claas had a big push on introducing shredlage to the market. One thing that stuck with those who attempted it, is that kernels are crushed or ground as much as possible. While the debate on longer chop lengths goes back and forth, the level of shredding from the special crop processor in Claas foragers has made everyone aware of the so-called Corn Silage Processing Score or CSPS for short.
Some farms will do checks using shaker boxes to asses the quality of the chopped material, but, on many more farms, silage is only analysed at the lab once harvest is complete. But then it’s too late to make any adjustments to the forager that could have improved the quality.
Analysis in the field
Claas is now going one step farther and providing contractors and customers with a very interesting tool — a CSPS assessment app that analyses the chopped material in a few minutes … and it can do this in the field. This chop quality analysis developed by Claas is based on artificial intelligence (AI), which scans photos of chopped material, identifies kernel fragments and determines their sizes. The photo analysis AI has been trained by a team of developers led by Sven Belau whose aim was to create a simple and practical tool that’s able to check a forager’s processor performance in the field.
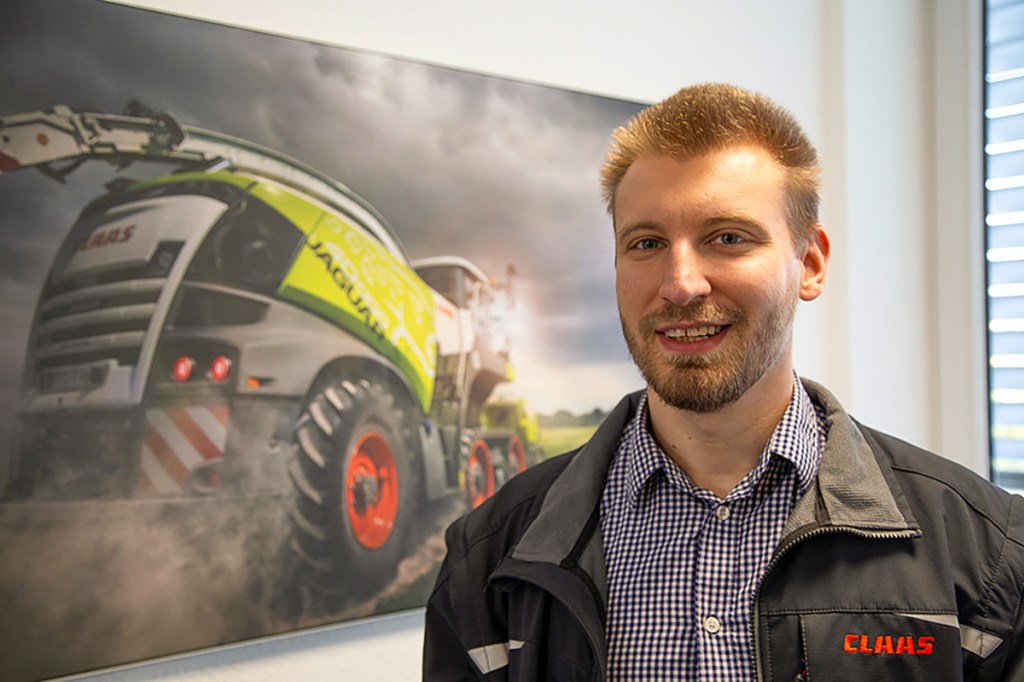
Previous systems, such as Silage Snap (an app from America), didn’t quite achieve the user friendliness Claas wanted, because the chopped sample has to be dried before it can be measured. In addition, a reference size must be placed in front of the camera, similar to an X-ray image.
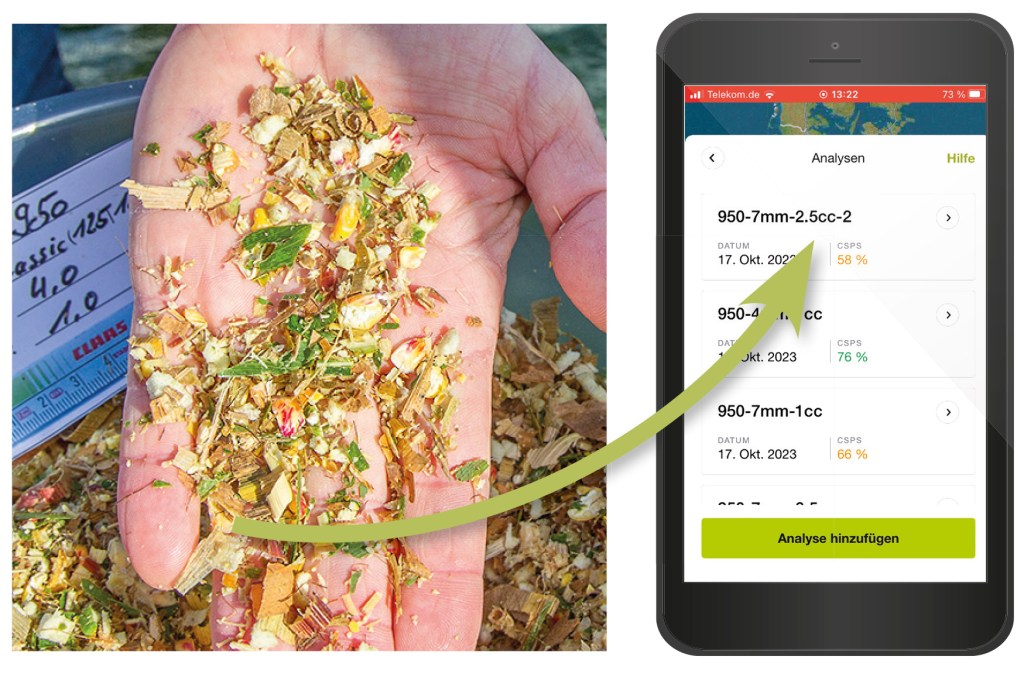
Blue plate
The chop quality analysis on the smartphone app in Claas Connect 3.0 uses a blue plastic tray that is no larger than an A4 sheet of paper and has a rough surface. This does not reflect as the photos are taken and can be simply stowed away in the harvester cab. The tray not only serves as a size reference, but the system can also use the rectangular form to correct the perspective of the image, because it’s not always possible to have the camera in exactly the same position as you take the snap.
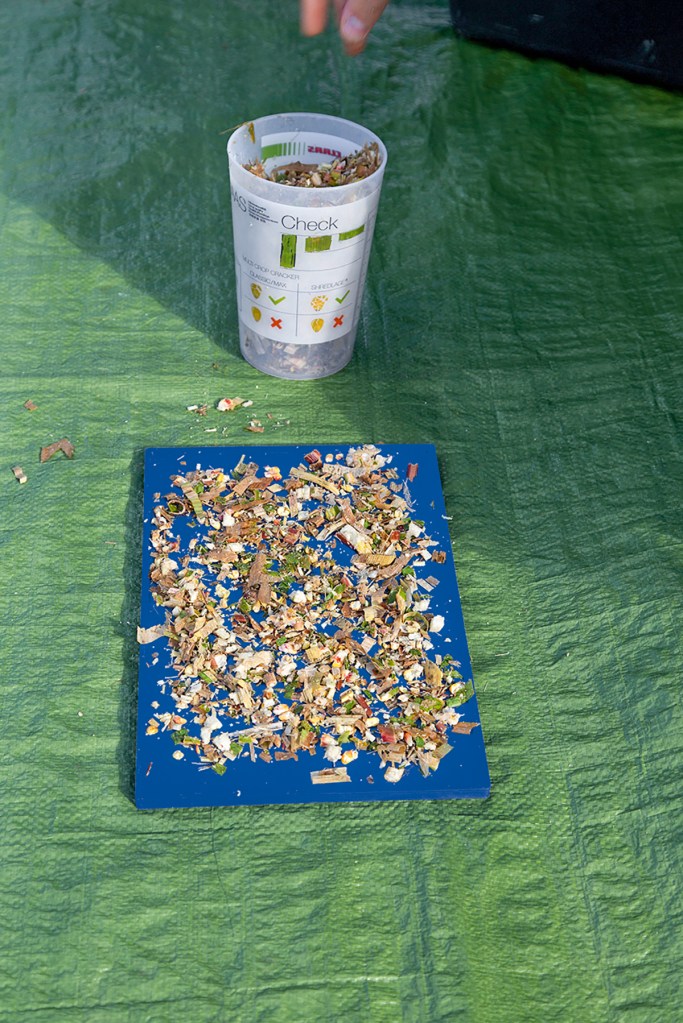
The usual good photography rules apply: the camera lens should be clean and the maize sample on the blue tray should be in focus. And, lastly, the edges of the blue tray must be visible in the photo. The picture quality from a regular smartphone is sufficient.
Once you have the photos, you then need to upload them direct from the mobile phone to the Claas Cloud. It is not possible to take photos of crop samples that are shown on another mobile phone or tablet, nor is it possible to upload scans of print images. Because the Claas system filters out poor or unusable photos immediately after upload, the photo quality is always up to the mark — in fact, there’s no need for users to think too much about it.
Minimum of six photos
In practice, at least six photos or samples should be uploaded for each chopper setting (cut length and cracker gap). To do this, take a handful of the freshly chopped material, spread it out loosely on the blue tray and take a photo — that’s it.
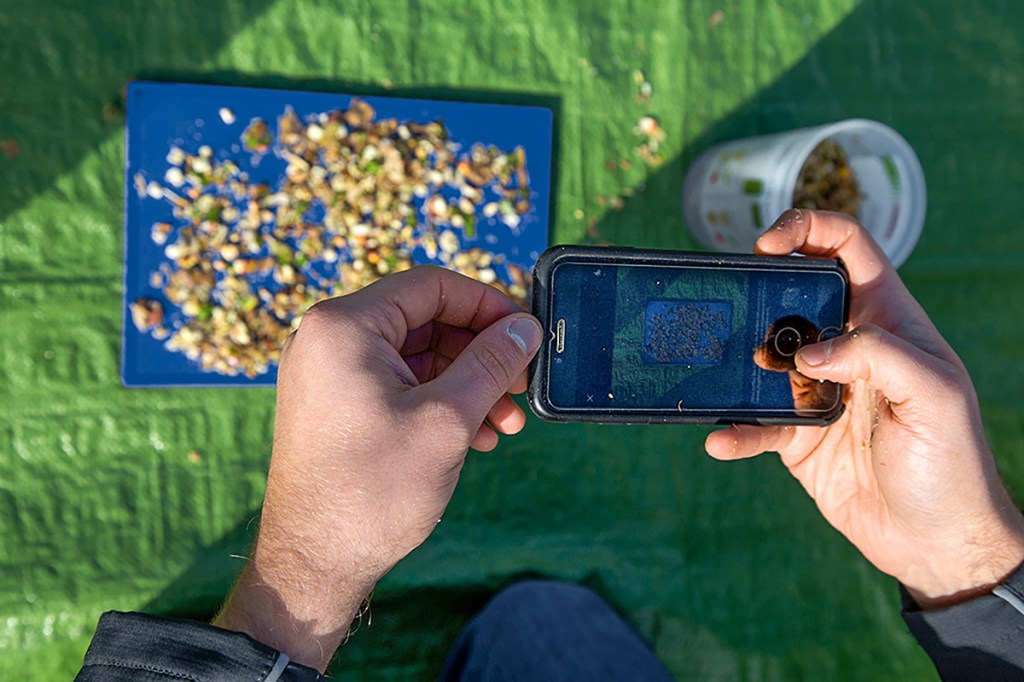
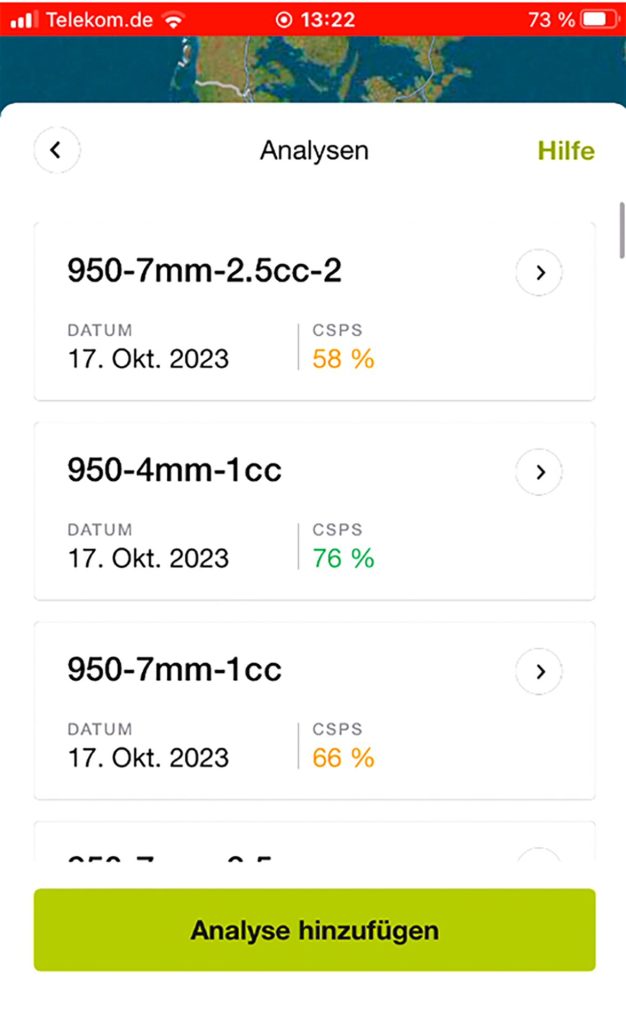
The photos can then be uploaded to the Claas cloud, where the system assigns them a sample number and evaluates them. This means that your mobile must be connected to the Internet, which as we know is not always possible; there are always areas with no reception — and if you’re uploading more than 50MB when there is poor network… good luck with that.
On the positive side, the photos are always saved in the Claas app so that they can be automatically uploaded and analysed once there is a good network connection — great.
To make a representative statement, the artificial intelligence should have recognised at least 300 kernel fragments in the photo. According to analyses by Claas, the statistical coefficient of determination is then already around 75%.
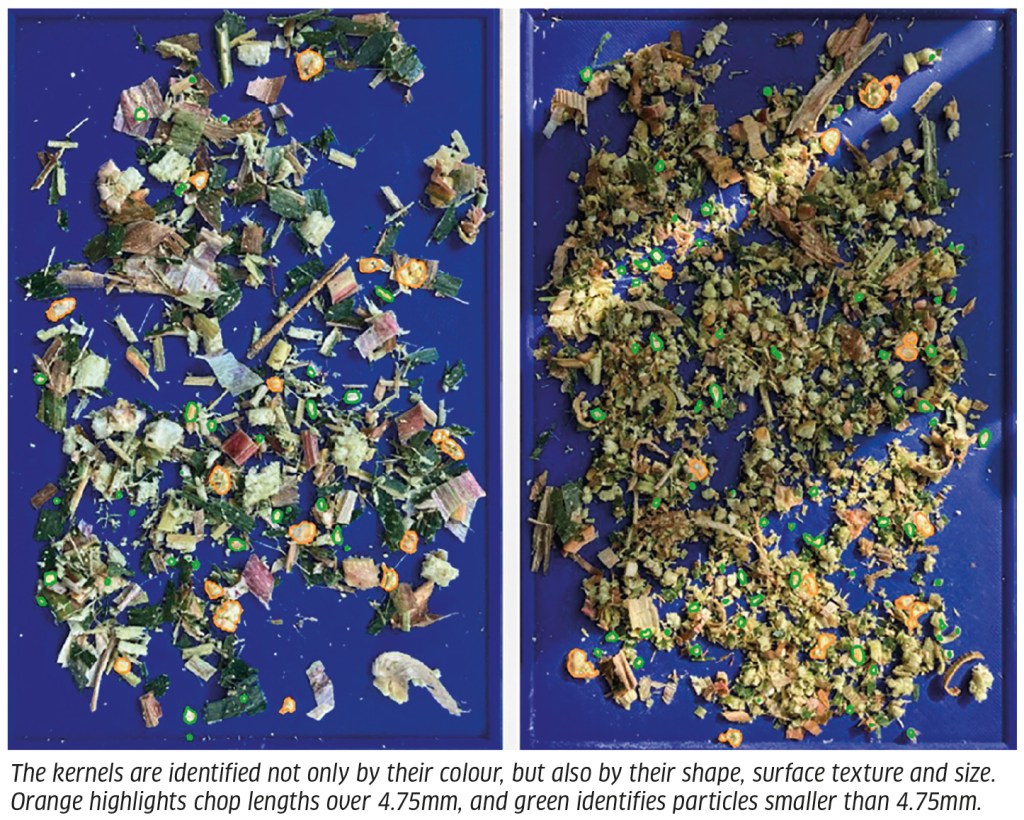
Since the size of the plastic tray is known, the technology can determine the size of the kernels (millimetres per pixel) and sort the fragments into coarse fraction (>4.75mm) and a fine fraction (<4.75mm). Based on the statistical frequency of certain sizes, the system then evaluates and determines CSPS content and gives the user an indication of the chopping quality on a coloured scale. Red indicates the CSPS is less than 50%, which is poor. Average quality is shown as yellow and corresponds to a CSPS score of 50-70%. Green is when the CSPS value is over the 70% mark.
This handy technology doesn’t measure but evaluates, because a lab sample will still be more accurate. This is because the system can’t recognise starch flour or tiny particles in the photo if they’re smaller than an image pixel. In this case, the starch content of the analysis is important in order to determine the actual CSPS content. Nevertheless, Claas states from its own experience that even the results of various labs analysing identical samples may fluctuate within a +/-5% range. This experience has been gained by training the AI over the past few years.
Comparison of field data with lab analysis results
We were able to test the system last year with samples produced by a Claas Jaguar. We compared the chop quality scores with lab results (Lufa Münster).
After we uploaded the photos, the results were returned to our phone in no time. We tested two chop lengths: 4mm and 7mm and increased the cracker gap from 1.0 to 2.5mm in another sample.
The Claas app hit two of the lab CSPS scores with decent accuracy. (See the table ‘Chop samples compared’). The dry matter content varied between 37% and 43% for the same maize variety.
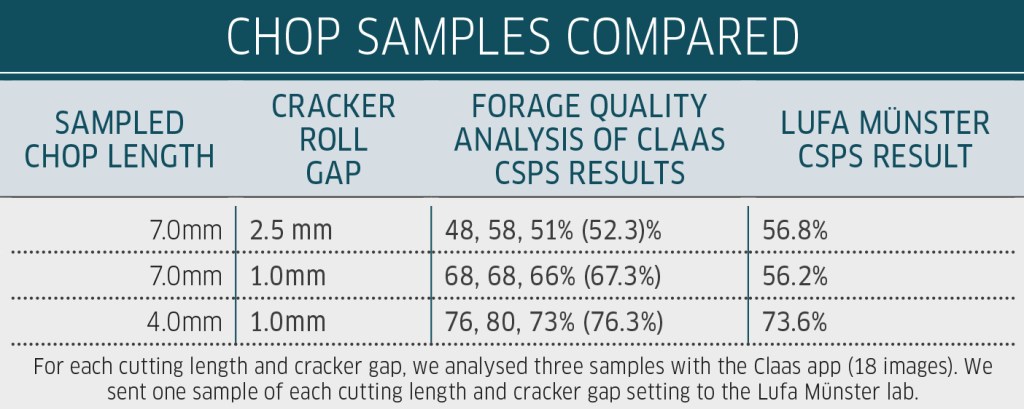
In light of the lab fluctuations mentioned above and the fact that Claas categorises AI as an evaluation tool, we were pleased with the results. Remember that the AI learns automatically and thus improves accuracy as the number of samples increases. Claas is convinced of this.
Further details
- The new Claas application is initially only available to Claas customers. The service costs £950.
- Being able to quickly analyse the level of crop shredding is just the beginning. The Claas system will also be able to analyse the cutting quality of grass silage in the future.
- This, in turn, could allow the forager to automatically alter settings. And this is certainly just the beginning of an even “smarter” machine.
- It is not possible to upload photos from your own photo gallery.
- According to Claas technical staff, the coefficient of determination and thus the statistical accuracy is around 95%.
Summary
Claas’s forage quality analysis is a great example of how AI is finding its way into our everyday lives. Chop quality can be assessed and documented from a smartphone. And it is a straightforward process — chop samples are laid out on a blue tray and photographed. These photos are then uploaded to the Claas server where AI evaluation takes place in seconds.
Our first impression is promising, even if the lab analyses were not accurate. The system is just the beginning of self-adjusting forage harvesters — if you want them to be.
Tobias Bensing
For more up-to-date farming news click here and subscribe now to profi and save.